ABOUT THE JOURNAL
The Journal of Public Health in Africa is a peer-reviewed, academic journal that focuses on health issues in the African continent. The journal editors seek high quality original articles on public health-related issues, reviews, comments and more. The aim of the journal is to move public health discourse from the background to the forefront. The success of Africa’s struggle against disease depends on public health approaches. View our Editorial Team.
The Journal of Public Health in Africa is a peer-reviewed, academic journal that focuses on health issues in the African continent. The journal editors seek high quality original articles on public health-related issues, reviews, comments and more. The aim of the journal is to move public health discourse from the background to the forefront. The success of Africa’s struggle against disease depends on public health approaches. View our Editorial Team.
Quick links:

More Latest Articles... |
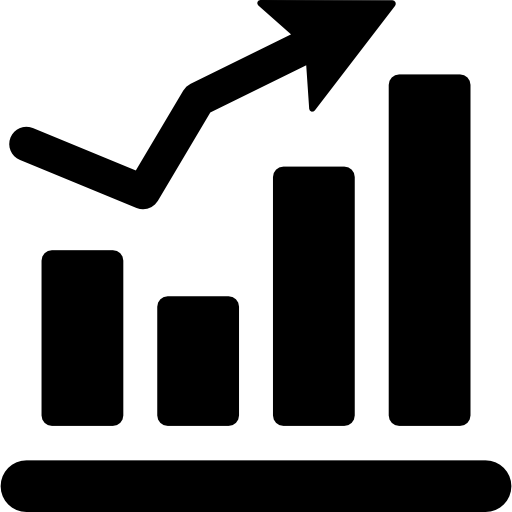
412
PUBLISHED
CONTENT
CONTENT
0
CROSSREF
CITATIONS
CITATIONS
5412
TOTAL
DOWNLOADS
DOWNLOADS

Press Release: Online Resource & News Portal - Department of Health, Republic of South Africa |
|
Visit www.sacoronavirus.co.za for up to date information about the COVID-19 Pandemic. Hotline: 0800 029 999 | WhatsApp: 0600-123456 | |
Posted: 2024-04-04 | |
Notification: The 2nd Declaration of Marrakech has been published |
|
We are thrilled to announce the publication of the 2nd Declaration of Marrakech, resulting from the 2nd African and 1st South-South Health Harm Reduction Conference. This significant event took place in the city of Marrakech from September 27th to 29th, 2023. With 83 participating countries, approximately 700 speakers, and more than 2,500 participants from all corners of Africa, the Global South, and the rest of the world, this conference was a resounding success. During this momentous gathering, the conference featured five panel sessions, two side events, five book signing sessions, two press conferences, and an exhibition area with over 45 exhibitors. The event was inaugurated by the esteemed presence of the Head of the Moroccan Government, Mr. Aziz Akhannouch, who delivered the opening remarks. Additionally, Ministers and Ambassadors from Africa, South America, Asia, and many other regions lent their support and insights to the conference. Under the overarching theme of "Health in Africa: Water, Environment, and Food Security," the conference provided a platform for meaningful discussions and exchanges of ideas. The collective expertise of distinguished speakers and participants enriched these discussions, shaping the outcome of the Declaration of Marrakech. | |
Posted: 2024-03-11 | More... |
More Announcements... |
ADVERTISEMENT